Reconstruction of Mastectomy Cases in Texture Space
You can view the shortened version of the thesis here
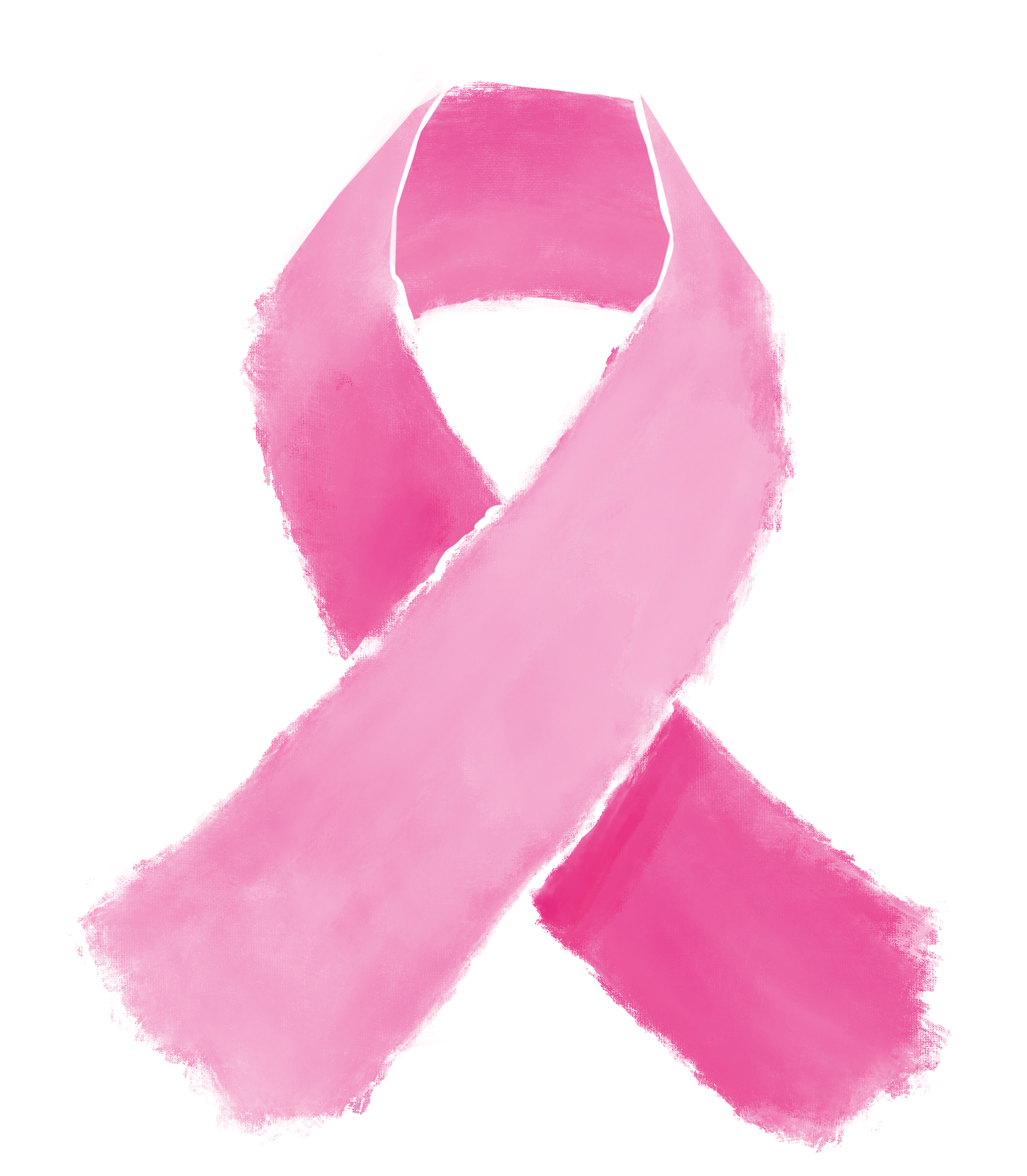
One in eight women will develop breast cancer in their lifetime. Treatment options include a mastectomy, which involves the removal of one or both breasts depending on the severity of the cancer. Scarring and skin folds may result from the procedure. Reconstructive surgery is an option, but predicting the outcome can be difficult. This thesis aims to create a pipeline to show women a possible outcome.
The research began with a literature review to build a foundational understanding. A comprehensive overview of the medical aspects was obtained, including an analysis of the various types of mastectomies and an overview of the potential applications of machine learning in the medical field. Segmentation of medical images is a common problem, yet no prior work has been identified that detects mastectomy scars or skin folds. Inpainting is a prevalent challenge in the domain of visual computing. While numerous models have demonstrated the capability to inpaint images of faces or locations, the same is not yet true for skin parts in the torso region.
The first stage of the implementation focused on segmenting skin folds and scars in mastectomy cases, involving research into suitable architectures, image collection, and manual annotation for model training. Machine-learning models were then trained to segment these features accurately. Next, a skin inpainting technique was implemented to replace the previously segmented skin folds and scars with normal skin. Furthermore, the implementation of inpainting techniques to restore missing nipple textures was explored.
The realism of inpainting was evaluated qualitatively using a test set with various scenarios, including different scar sizes and different amounts of skin folds. The primary goal was to create a pipeline capable of automatically detecting and removing scars and skin folds, providing patients with visual representations of possible reconstructive outcomes, including nipple reconstruction or tattoos. A survey was conducted to evaluate the effectiveness of the generated images. It assessed satisfaction levels, the ability to distinguish between edited and original images, and the aesthetic quality of the generated versus real nipples. Results showed that 71.96% of participants found the generated nipples more aesthetically pleasing than the original.